Disease Diagnostics Using Machine Learning of B Cell and T Cell Receptor Sequences
Machine learning (ML) has become an invaluable tool in healthcare, particularly for disease diagnostics. In recent years, researchers have focused on using ML techniques to analyze B cell and T cell receptor sequences, which are crucial components of the immune system. These receptors are responsible for recognizing pathogens and triggering immune responses. By utilizing advanced ML algorithms, scientists are improving the accuracy and efficiency of diagnosing diseases, such as autoimmune disorders, cancers, and infectious diseases.
Understanding B Cell and T Cell Receptor Sequences
B cells and T cells play a central role in the body’s immune response. B cells produce antibodies, while T cells help recognize and destroy infected cells. The receptors on both types of cells are highly specific to the pathogens they target. By sequencing these receptors, we can gain valuable insights into the immune system’s functioning and its response to diseases.
For more information on immune system functions, check out this research article.
Machine Learning in Disease Diagnostics
Machine learning algorithms, such as neural networks and random forests, are increasingly used to analyze B cell and T cell receptor sequences. These models can identify patterns in the sequences that indicate the presence of certain diseases. For example, they can differentiate between healthy immune responses and those triggered by cancerous cells or viral infections. This approach significantly reduces the time it takes to diagnose complex diseases, leading to earlier intervention and better patient outcomes.
Learn more about machine learning in healthcare.
Benefits of Using ML for Receptor Sequence Analysis
By applying machine learning to the analysis of receptor sequences, researchers can discover new biomarkers for disease detection. This technology not only enhances diagnostic accuracy but also provides a deeper understanding of how the immune system responds to various conditions. Furthermore, the use of ML in this area allows for the analysis of vast amounts of sequencing data, which would be impossible to manage manually.
Discover more about the potential of machine learning in biotechnology.
Real-World Applications of ML in Disease Diagnostics
Machine learning’s application in disease diagnostics has already shown promising results. In cancer detection, ML models can analyze T cell receptor sequences to identify mutations that may indicate the presence of tumors. Similarly, in autoimmune diseases, ML can pinpoint the genetic markers associated with conditions like rheumatoid arthritis and multiple sclerosis.
To understand more about the real-world impact of ML in diagnostics, visit this resource.
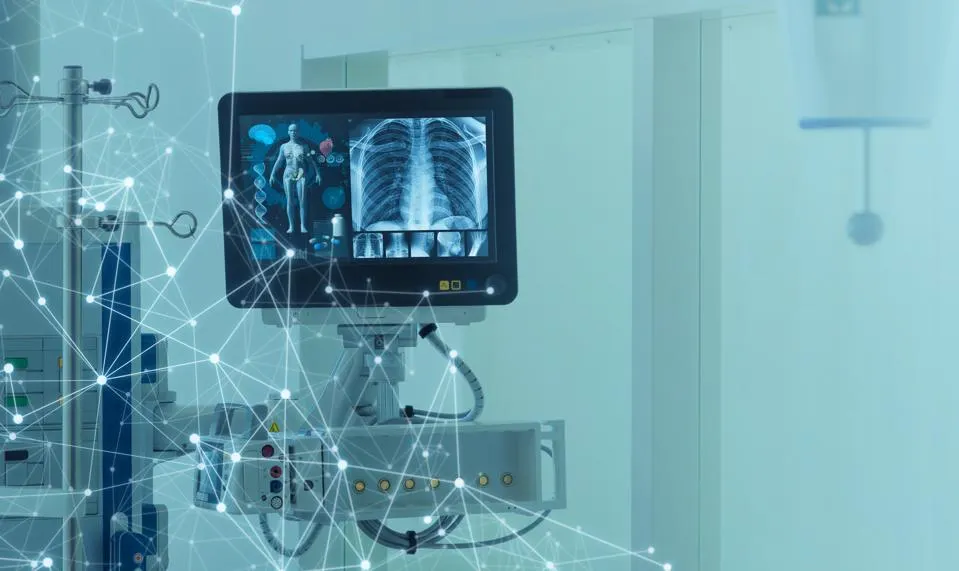
Challenges and Future Directions
While the use of ML in disease diagnostics is revolutionary, it does come with challenges. The quality of sequencing data is essential, as errors or inconsistencies can lead to inaccurate diagnoses. Additionally, the complexity of immune system responses means that models need to be constantly updated and refined to account for new findings. Despite these challenges, the future of ML in disease diagnostics looks promising, with ongoing advancements in both technology and healthcare applications.
For insights into overcoming these challenges, explore this article on ML in healthcare.
Conclusion
Machine learning has the potential to revolutionize disease diagnostics by analyzing B cell and T cell receptor sequences. With its ability to uncover hidden patterns and improve diagnostic accuracy, ML is enabling faster, more efficient detection of diseases. As research continues to evolve, we can expect machine learning to play a crucial role in advancing healthcare diagnostics.
Explore more about advanced machine learning techniques for disease detection.
for more details of machine learning: